AI Theory, Foundations, Methods, and Systems
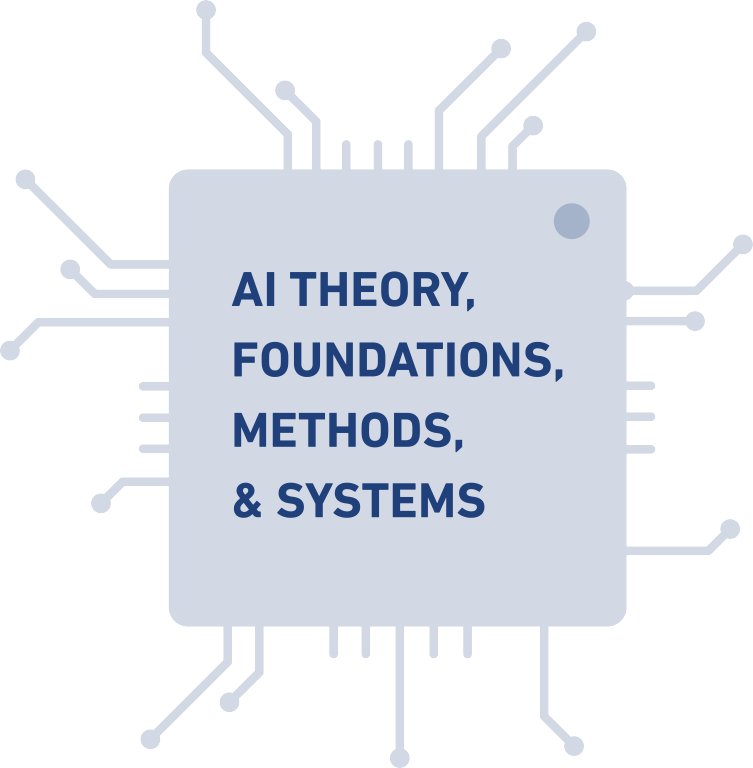
Emerging applications and the big challenges of our time call for new fundamental research in AI. In this research area, we focus on new methodological developments and mathematical theory that will impact the upcoming decades.
For instance, we need learning methods with improved efficiency in terms of data and computation, models that robustly adapt to changing environments, safe and explainable AI systems, and active learning strategies to overcome limitations of current predominately passive approaches.
In addition to theoretical and statistical foundations, our expertise covers new fundamentals in algorithm and model design for machine learning, as well as their integration in intelligent systems — ranging from small, highly power-constrained hardware to large-scale data centers and cloud-based platforms.
To advance the foundations, we team up across departments and disciplines. This was already recognized early on with ETH’s Foundations of Data Science (FDS) program that brings together researchers of the departments of Mathematics (D-MATH), Computer Science (D-INFK), and Information Technology and Electrical Engineering (D-ITET). With the launch of the ETH AI Center, the community on AI foundations has now further evolved into a group of Professors from eight different departments.
Research topics include
- Mathematical and Statistical Foundations of Machine Learning
- Interpretability and Explainability
- Safety, Reliability, and Robustness
- Fairness and Bias of AI Systems
- Machine Perception
- Natural Language Understanding
- Reinforcement Learning and Data-driven Control
- Neuroscientific Foundations
- AI Edge and Networked Computing
- Privacy-Preserving AI Systems
- AI System Design and Engineering