Machine learning for fusion research
Date: Tuesday, 26 April, 17:00-18:00
Speaker: Dr Alessandro Pau
Location: Join in HG D7.2 or via external page Zoom
Speaker Bio
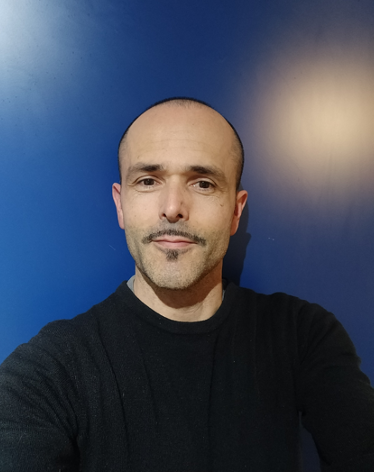
Alessandro Pau is a Research Scientist at the Swiss Plasma Center (EPFL) in Lausanne. He is actively involved in various fusion research activities in the framework of the EUROfusion Research Programme and the International Tokamak Physics Activity (ITPA), where he coordinates high-level research topics concerning critical issues in tokamak physics and plasma control. His research, currently, focuses on understanding the complex nonlinear dynamics characterizing the temporal evolution of magnetic confined plasmas in Tokamaks, with particular reference to the catastrophic loss of plasma thermal confinement known as disruption, which needs to be avoided to preserve the integrity of fusion devices and to enable the control of stable and high-performance plasma regimes. He is responsible for several collaborations and projects concerning the exploitation of AI and machine learning in fusion research. In this context, he is currently focusing on the development of tools to enhance model’s “explainability”, with the aim of improving the understanding of the complex physics mechanisms leading to the loss of plasma control and disruptions (“data-enhanced physics informed predictions”).
Abstract
Nuclear fusion based on magnetic confinement, such as in the tokamak configuration, represents one of the most promising paths towards fusion energy, and this latter, in turn, represents one of the most accredited alternatives for the generation of sustainable electric power. Among the various challenges fusion research is currently facing, the control of stable, high-performance plasmas in a tokamak has proven to be extremely difficult and is object of many experiments in the present fusion devices in the view of ITER, the largest magnetic confinement experiment based on the tokamak concept, which is being built in Cadarache, in the south of France. In this context, AI and machine learning offer several tools to automatize, scale-up and speed-up computational demanding, complex data processing tasks. The massive volume of high-dimensional data collected with the various diagnostic systems poses several challenges, which are common to other complex real-world systems too. Data from fusion experiments are extremely heterogenous, acquired in very different conditions, and present multiple timescales. From a physics and control point of view, one of key goals is to understand the complicated patterns in plasma state dynamics in order of being able to optimize plasma performance while preventing the system from evolving towards operational and stability boundaries, where tokamak plasmas can undergo a "catastrophic" loss of thermal confinement known as disruption. With reference to this specific context, this talk will discuss how machine learning and AI systems can support fusion research, enabling and accelerating the progress toward the realization of fusion energy.